Train companies are moving away from time-based maintenance to a more predictive paradigm.
Window of opportunity: time vs condition-based maintenance
Traditional time-based maintenance (TBM), and reactive ‘fail and fix’ or planned maintenance practices, can be costly, prone to human error, or lead to downtime or accidents. Train companies are understandably moving away from TBM in increasing numbers in favour of condition-based maintenance (CBM), in which servicing of machinery is performed when the need arises.
Raghavan uses an analogy of a car in order to illustrate the concept of CBM. A car needs an oil change every six to 12 months or 5,000–10,000 miles. Newer models have sensors that tell the owner when the service is due based on average usage time, but this may not take into account factors such as heavy loads or frequent use. The service schedule may be too conservative, for example.
The worst that can happen, of course, is that the car stops working, but extrapolate that out to a large complex asset such as a train and the problem immediately becomes much more significant.
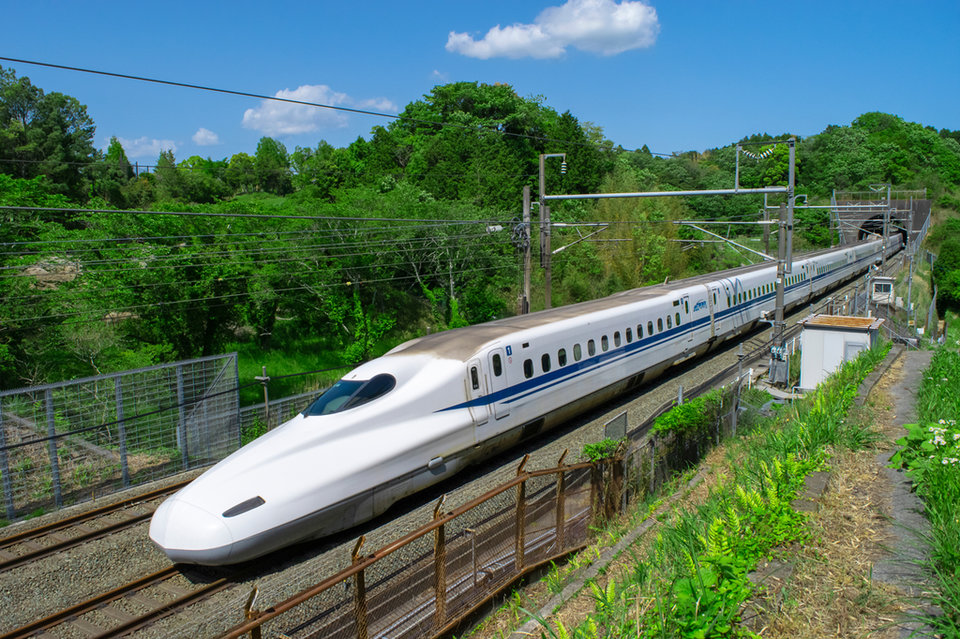
Image: tackune | Shutterstock
“The traffic in the Tokyo subway system is crazy, especially during peak hours, and if a train stops working the entire system clogs up, or in the most extreme cases accidents can result in loss of life with operators liable,” says Raghavan. “TBM may have worked for assets that have similar usage patterns, but not necessarily for those with a significant geographical or climate spread, or those stretched beyond their original design life; in these instances TBM practices are no longer cutting it.
“Train companies are therefore moving away from TBM to this more predictive paradigm where O&M teams may get a week or even a month’s notice before assets fail, allowing them to prepare schedules and resources to avoid taking a critical train out of service and disrupting the system.
“Predictive analytics is also extremely valuable in terms of long-term planning. Providing operators with data on the remaining useful life of an asset one or even six months before it has to be retired enables them to plan ahead for a major capital outlay, for example.”
The MOXI™ technology suite explained
JR East had collected a significant amount of lab and field data, but were struggling to understand it.
“They were getting 70%–80% success but not enough for the operations and maintenance teams to be sufficiently confident with the capabilities,” says Raghavan. “They were also sceptical about CBM.”
PARC deployed its MOXI™ technology suite, which utilises model-based algorithms that enable 90% or higher accuracy and low false alarm rates, and in some cases require only minimal data sets.
“The first system we explored was train doors which, in the UK, can cause as much as 30% of all delays,” explains Raghavan. “There is a lot of them and they are used every time someone uses the subway or other major transit trains. Doors are supposed to work 100% autonomously and they do a reasonable job most of the time, despite being subjected to quite enormous loads, but it only takes one to fail to take the entire train out of operation.
“So this was of huge importance to JR East because the company prides itself on being on time, all the time. Within a matter of five months our PARC team was able to demonstrate first proof of concept of our predictive CBM working for the train door system with 95% accuracy or higher in classifying the different types of faults and, thanks to our algorithms, very low to minimum false alarm rates.”
Dashboards were also developed to enable JR East engineers to visualise and better understand the obtained data. PARC and its Asian consultancy partner NRI are now working with JR East Technical Centre teams to test and implement these solutions on the heavily used Yamaman rail line.
“We have also built a computerised analytics solution that automatically analyses the images taken by Solus trains that operate on tracks overnight,” adds Raghavan. “Our algorithms make sense of them and identify different types of faults.”
PARC’s condition-based maintenance worked for the train door system with 95% accuracy.